EXPERT IN THE SPOTLIGHT: Teodora Savic Popovic is a professional data engineer, PhD student in applied mathematics at the University of Novi Sad, Serbia, and an enthusiastic cyclist. She is profiled in the domain of telecommunication and supply chain data management and machine learning and she offers insights into the critical role of data engineering and its impact on modern businesses.
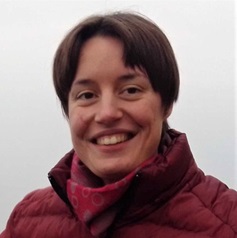
TOPIC IN THE SPOTLIGHT: In the world of data-driven decision-making, the role of data engineers is ever increasing. These professionals are the architects behind the scenes, responsible for constructing the frameworks that process, manage, and organize vast amounts of data. Their expertise lies in transforming raw data into meaningful insights, thereby enabling businesses to make informed decisions. In this interview we explore intricacies, challenges, and the captivating stories behind the scenes of data engineering.
With a diverse expertise portfolio, our guest today, Teodora Savic Popovic, will share intriguing anecdotes and provide the most recent insights in the data engineering and data management field.
To summarize things shortly: I work in the IT industry as a data engineer, with experience ranging from telecommunications to supply chain in the food production industry, also I am a PhD student in Applied Mathematics at the University of Novi Sad in Serbia.
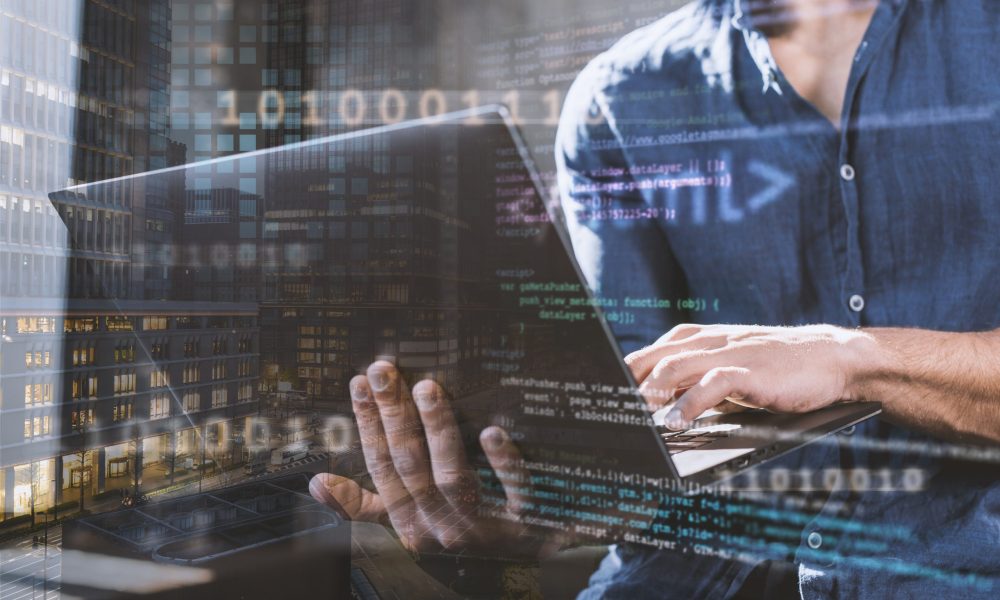
Of course! So, like I have mentioned in our small talk, to have a well-defined data flow is essential, and well-defined data flow certainly does not tolerate duplicates. Anyway, at my desk the following task was dropped: find why the table that marks load delivery has duplicates. And really, for one driver we had duplicate deliveries for the same load. Of course, I have made a lineage of data from its entering to data staging area until it’s export to the DWH and business reports. And I could find neither one logical mistake.
In the call with the client, I described him the issue. And after some time thinking about it, he shouted: “Wait a minute! Read me again the driver’s ID and name. I think I know what is in between! I know who the driver is – the old Joe is in his 70ties, for surely he was practicing how to use the application in which he must mark the load delivery, so probably while exploring the application, he entered the same load twice!” So, anyway, sometimes the unclean data could tell a real-life story of its contributors.
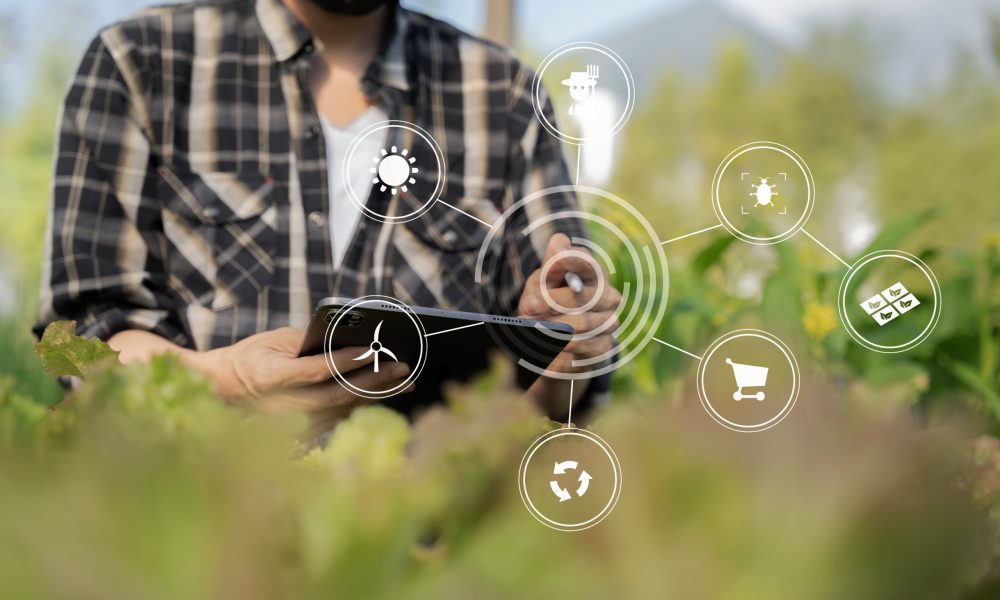
Well, the data science, AI and ML really can be found in every soup today. But, just to make it clear, there is no efficient machine learning without clean and reliable data. You must have clean data on which you want to test your machine learning model, you want to avoid the scenario: garbage in, garbage out. And to do so, you must have well-structured data, and well-structured data comes with good data preparations and engineering. I will put it this way: First on the plate comes data engineering, then for the desert we have machine learning.
First on the plate comes data engineering, then for the desert we have machine learning.
Teodora Savic Popovic Tweet
Well, the data issues manifest in various forms. But let’s just mention a few of them. For example, discrepancies arising from faulty ETL (Extract, Transform, Load) logic pose significant challenges for both data engineers and business analysts. Also, erroneous data due to user input errors adds complexity to data processing (have in mind the old Joe’s scenario with his duplicates loads).
Final thoughts
So, at the end of the story we can conclude the following – for a successful project, it is essential to have a good collaboration between all players involved in it, to focus on data preparation and cleaning, to build efficient data pipelines that are based on business logic to deliver reliable reports. In CrackSense, consortium partners with overlapping but distinguished areas of expertise join forces and explore the possibilities of utilizing different data collection and sensory tools as well as differentiating an efficient, effective and insightful data pipeline systems to combat fruit cracking.